Jason Hartford
Dame Kathleen Ollerenshaw Fellow at the University of Manchester (starting October 2024), and Research Unit Lead / Staff Research Scientist at Valence Labs.
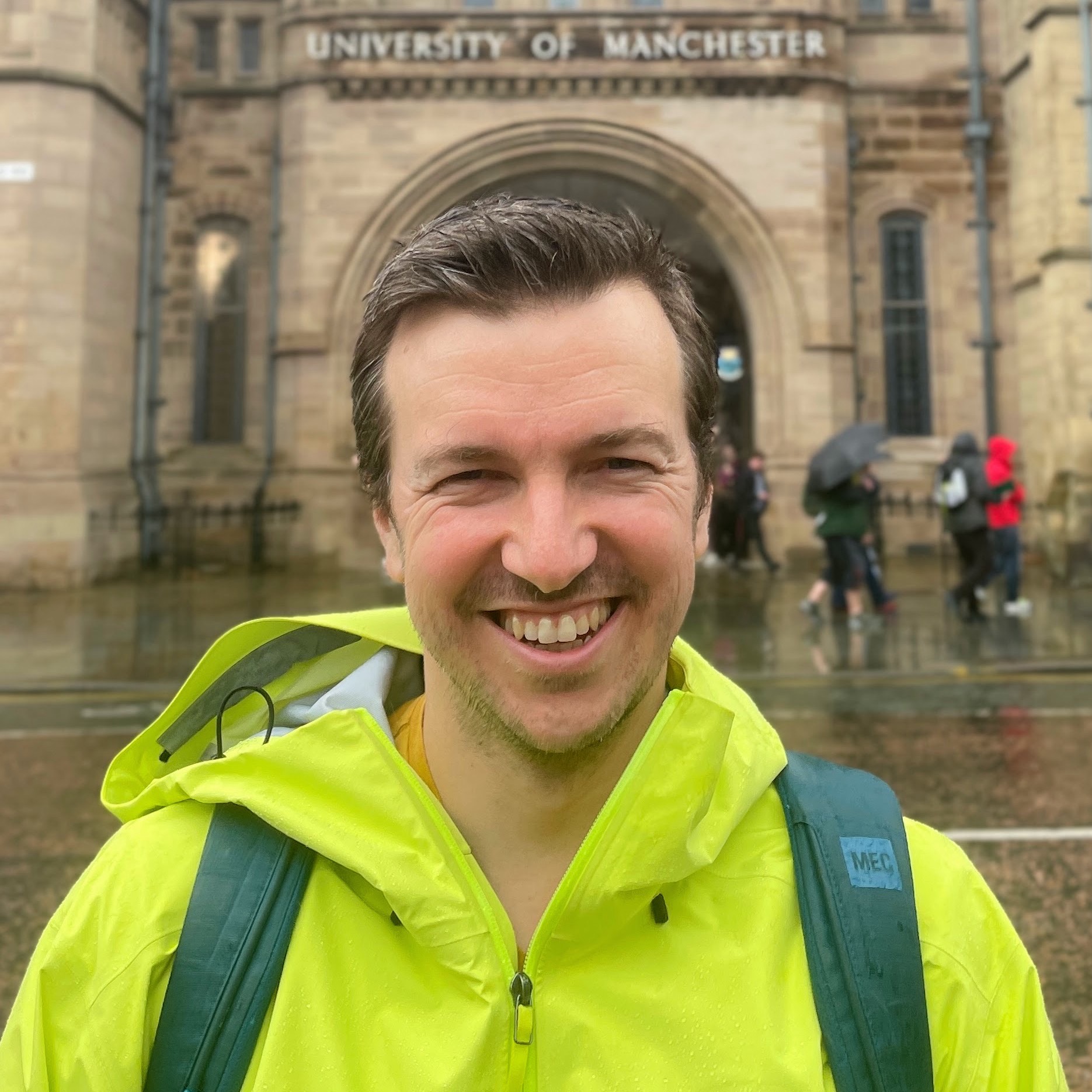
I work on building the tools to enable scientific discovery from complex, high-dimensional data. Most of my current research focuses on developing new techniques for causal representation learning and causal inference, as well as active learning techniques for designing experiments to learn causal models. Before starting at Valence and Manchester, I completed a post-doc with Yoshua Bengio at Université de Montréal, and before that, I completed my PhD at the University of British Columbia with Kevin Leyton-Brown.
I am looking for PhD students to work on these problems. Please get in touch if you are interested! I also have two CDT funded positions available on causal abstraction of dynamical systems and unpaired multimodal representation learning (deadline 31 January 2025).
news
Dec 19, 2024 | I am co-organising the Learning Meaningful Representations of Life workshop at ICLR 2025. |
---|---|
Dec 1, 2024 | We had two papers in the main conference (Targeted Sequential Indirect Experiment Design and Propensity Score Alignment of Unpaired Multimodal Data) and three in the workshops (foundation models, mechanistic interpretability, and score-based interaction testing) at NeurIPS 2024! |
Dec 1, 2024 | Our paper on large-scale masked autoencoders for cellular microscopy received the runner up best paper award at the NeurIPS 2024 Workshop on Foundation Models for Science. |
Dec 1, 2024 | I gave an oral presentation on our dictionary learning paper in the NeurIPS 2024 Workshop Interpretable AI workshop. |
Oct 19, 2024 | I now an Ellis member and can supervise students through the Ellis PhD program. |